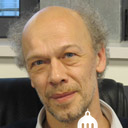
Team : MLIA - Machine Learning and Information Access
Axe : .Team leader :
Patrick Gallinari
Short presentation
The MLIA team left LIP6 at the end of 2021.
Team website: here
The central topic of the team is statistical machine learning with an emphasis on algorithmic aspects and on application domains involving semantic data analysis. The mains axes of our fundamental research contributions are:
- Representation learning and Deep Learning: representation learning emerged over the last ten years at the confluence of different domains with the aim of learning meaningful representations from data. The flagship today is represented by Deep Neural Networks. MLIA has a strong historical position on this domain and a large part of its research is within this field. Over this period, we have investigated learning representation for structured and dynamic data, with applications in Computer Vision, Natural Language Processing, Social Data Analysis and Recommendation.
- Structured outputs: learning to produce complex outputs encompasses many fundamental and application problems. A research direction has been Extreme Classification, a new challenge consisting in classifying with millions of classes. MLIA has organized 4 international challenges and workshops on this topic and participated to a EU project. We are also investigating classification in graphs for social networks and image segmentation. In a Franco-Canadian project, we are combining deep learning and structured prediction.
- Sequential and Reinforcement learning: Many machine learning problems can be revisited as sequential learning problems. This allows one to define new learning methods, and to extend existing models to more complex problems. We have investigated the development of new sequential models for different key tasks like structured output prediction, graph processing, budgeted learning and attention models. We have organized one workshop on this topic at ICML 2013.
Theoretical and algorithmic contributions are often developed together with practical applications in selected domains at MLIA:
- Computer Vision: we have developed several models for visual pattern detection and recognition. We have explored together computer vision-based approaches, bio-inspired modeling, and deep learning strategies for many years. This gives to MLIA a strong position in the current era of deep convolutional neural nets.
- Natural Language Processing and Information Retrieval, Recommendation: we have developed models for joint review, text polarity and recommendation prediction.
- Complex data analysis: we have developed statistical models for the analysis and modeling of information diffusion on social net by formulating the problem in continuous spaces instead of discrete ones. We have also developed a cooperation with actors like Renault and Ile de France Transportations (STIF) for analyzing problems in the transportation domain.
Teaching
MLIA is strongly involved in the Data Science Master cursus launched in 2014, with 9 courses taught by team members, L. Denoyer is co-responsible for this cursus.
National/ International Collaborations
MLIA has developed close international cooperation with China, Brazil, Italy, Singapour involving co-publications and researcher exchanges. It participates to joint projects with different countries (Greece, Belgium, Italy, Germany, Canada, Saudi Arabia). In France MLIA has been collaborating with teams in Grenoble, Lille, St Etienne, Marseille. MLIA is engaged in 2 international and 3 national academic projects.
Cooperation with Industrial partners
We have been engaged together with the LFI team in a close cooperation with Thales embodied in a joint lab. for 6 years now. MLIA has several close cooperation with industrial partners, mostly through joint national or EU projects. MLIA participates to 1 international and 5 national R&D projects. On September 2016 MLIA was selected together with 22 European teams, as a recipient of the Facebook partnership program for providing academic labs GPU servers for promoting AI and Deep Learning.
Statistical machine Learning, Neural Networks, Deep Learning, Reinforcement Learning, Vision, Natural Language Processing and Information Retrieval
2013 January → 2021 December