Séminaire de l'équipe LFI
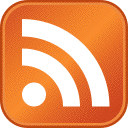
When factorization meets argumentation: towards argumentative explanations for recommendations
Jeudi 8 février 2024Jinfeng ZHONG (Université Paris Dauphine-PSL)
Résumé : Existing recommender systems frequently utilize factorization-based models, which are known for their efficiency in predicting ratings. However, the explicit semantics of the latent factors they learn are not always clear, complicating the task of explaining the recommendations. In contrast, argumentation-based methods have emerged as a significant tool in the realm of explainable artificial intelligence. In response, we propose a novel framework that synergizes factorization-based methods with argumentation frameworks (AFs). The integration of AFs provides clear semantics at each stage of the framework, enabling it to produce easily understandable explanations for its recommendations. In this framework, for every user-item interaction, an AF is defined in which the features of items are considered as arguments, and the users' ratings towards these features determine the strength of these arguments. This perspective allows our framework to treat feature attribution as a structured argumentation procedure, where each calculation is marked with explicit semantics, enhancing its inherent interpretability. Additionally, our framework seamlessly incorporates side information, such as user contexts, leading to more accurate predictions. We anticipate at least three practical applications for our framework: creating explanation templates, providing interactive explanations, and generating contrastive explanations. Through testing on real-world datasets, we have found that our framework, along with its variants, not only surpasses existing argumentation-based methods but also competes effectively with current context-free and context-aware methods.
Bio: I am a Temporary Lecturer and Research Assistant at Université Paris Dauphine-PSL. I successfully defended my Ph.D. thesis on the 30th of November 2023 (supervised by Elsa NEGRE). The focus of my thesis was Generality and Explainability in Recommender Systems, a topic that resonates within the field of artificial intelligence. Before joining LAMSADE for my doctoral studies, I pursued a Master’s degree at Université Paris Dauphine-PSL and completed my engineering education at AgroParisTech, under the guidance of Antoine CORNUEJOLS and Juliette Dibie. My academic path commenced with a Bachelor’s degree from Shanghai Jiao Tong University. My research interests are deeply rooted in artificial intelligence, focusing on general explainability and interpretability. This includes specialized areas like argumentation for explainable recommendations, counterfactual reasoning in the context of explainable recommendations, and methodologies for evaluating explanations.
Plus d'informations ici …
Christophe.Marsala (at) nulllip6.fr