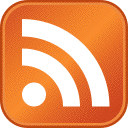
Demi-journée «Architectures IA accélérateurs matérielles et logiciels»
Tuesday, September 24, 2024L'axe ASR invite tous les membres intéressés à prendre part à sa demi-journée thématique «Architectures IA accélérateurs matérielles et logiciels». Trois exposés sont au programme, suivis par une table ronde et discussions.
Programme des présentations :
14h - 14h45 EdgeAI: Harnessing novel algorithms for Near-Sensor Intelligence - Paolo Meloni
Abstract : This talk explores the potential of EdgeAI, with a focus on innovative algorithms such as Spiking Neural Networks (SNNs) and Transformers. This presentation will delve into how SNNs, with their neuromorphic efficiency, and Transformers, with their powerful sequence modeling capabilities, are being adapted for edge deployment, using FPGAs and low-power microcontrollers as technology substrate. Several use-cases will be presented, mostly focusing on applications in wearable healthcare, showcasing fast, accurate, and energy-efficient biosignal processing directly at the edge.
Short-Bio : Paolo Meloni is an associate professor at the University of Cagliari. His research activity is on the development of advanced digital systems, on the application-driven design and programming of low-power processing platforms and FPGAs. He is the author of a multiple international research papers. He teaches Microcontroller-based systems and Advanced embedded systems at Università degli studi di Cagliari.
14h45 - 15h30 Design of Dependable Neuromorphic Computing Systems - Haralampos-G. Stratigopoulos
Abstract : Neuromorphic computing is an emerging computing paradigm aiming at solving various problems, such as visual sensing and perception, control-loops for robotics, brain-computer interfacing, audio processing, etc., with comparative advantage to the contemporary von Neumann computing architecture as well as the classical artificial neural networks. Neuromorphic computing has as basis a Spiking Neural Network (SNN) that emulates brain-like functionality. Input information is coded into spike trains and spikes, or events, propagate asynchronously through the network. This event-based operation as well as the sparsity of events enable a very low-power operation. Nowadays, there is intense activity in designing VLSI implementations of SNNs with some proprietary large-scale hardware platforms already available for research purposes. One may believe that SNNs inherit the remarkable fault tolerance of the biological brain. This is to some extend true, but recent fault injection experiments have shown that certain hardware-level faults can be critical. This talk will provide a review of the state-of-the-art of SNN VLSI implementations covering design but also dependability aspects, such as reliability, testing, and security. The talk will conclude with perspectives on this rapidly evolving field.
Short-Bio : Haralampos-G. Stratigopoulos received the Diploma degree in electrical and computer engineering from the National Technical University of Athens, Athens, Greece, in 2001, and the Ph.D. degree in electrical engineering from Yale University, New Haven, CT, USA, in 2006. In 2007 he became a Researcher with the French National Centre for Scientific Research (CNRS) at the TIMA Laboratory of the Université Grenoble Alpes, Grenoble, France, and in 2005 he moved to the LIP6 Laboratory of the Sorbonne Université, Paris, France. Since 2020 he is a Research Director with the CNRS at LIP6. His main research interests include hardware security, neuromorphic computing, and design-for-test for analog, mixed-signal, and RF circuits and systems.
15h30 - 16h15 Subspace Preserving Quantum Machine Learning Algorithms - Léo Monbroussou
Abstract : Current quantum devices have serious constraints, limiting the size of the circuits. Variational quantum algorithms, which use a classical optimizer to train a parametrized quantum circuit, have emerged as a leading strategy to address these constraints, and design powerfull quantum algorithms. Nevertheless, challenges remain including the trainability, accuracy, and efficiency of VQAs. Subspace preserving quantum circuits are a class of quantum algorithms that, relying on some symmetries in the computation, can offer theoretical guarantees on their training. Those models are sub-universal, and cannot offer an exponential advantage. In this talk, we will discuss how to use those methods to mimick classical neural network architectures, and how to seek potential advantages while using a particular quantum hardware.
Short-Bio: Léo Monbroussou is a third year PhD student at LIP6 in the Quantum Information team, and at Naval Group, under the supervision of Elham Kashefi and Alex B. Grilo. His recent works focus on assessing potential advantage in near term quantum machine learning in terms of expressivity, trainability, and data encoding, among others.